About AWS
Overview
Since launching in 2006, HAQM Web Services has been providing world-leading cloud technologies that help any organization and any individual build solutions to transform industries, communities, and lives for the better.
As part of HAQM, we strive to be Earth’s most customer-centric company. We work backwards from our customers’ problems to provide them with cloud infrastructure that meets their needs, so they can reinvent continuously and push through barriers of what people thought was possible.
Whether they are entrepreneurs launching new businesses, established companies reinventing themselves, non-profits working to advance their missions, or governments and cities seeking to serve their citizens more effectively—our customers trust AWS with their livelihoods, their goals, their ideas, and their data.
AWS is how
AWS helps organizations of every type, size, and industry innovate and transform their business in new and exciting ways.
Videos
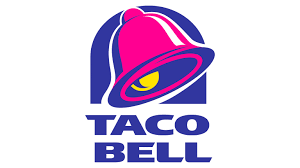
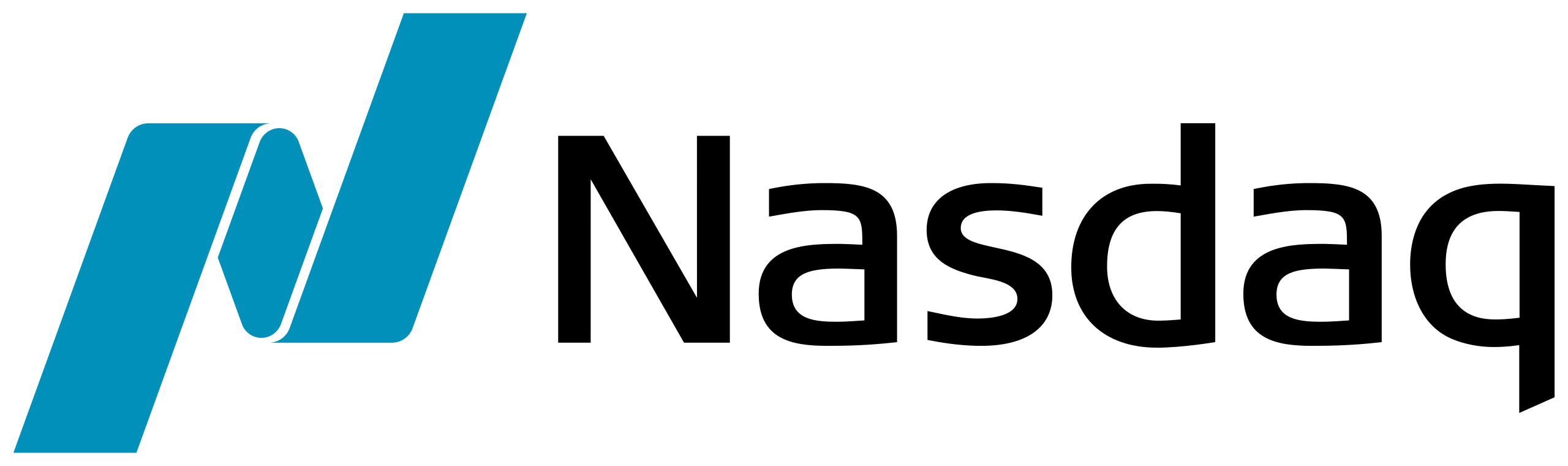
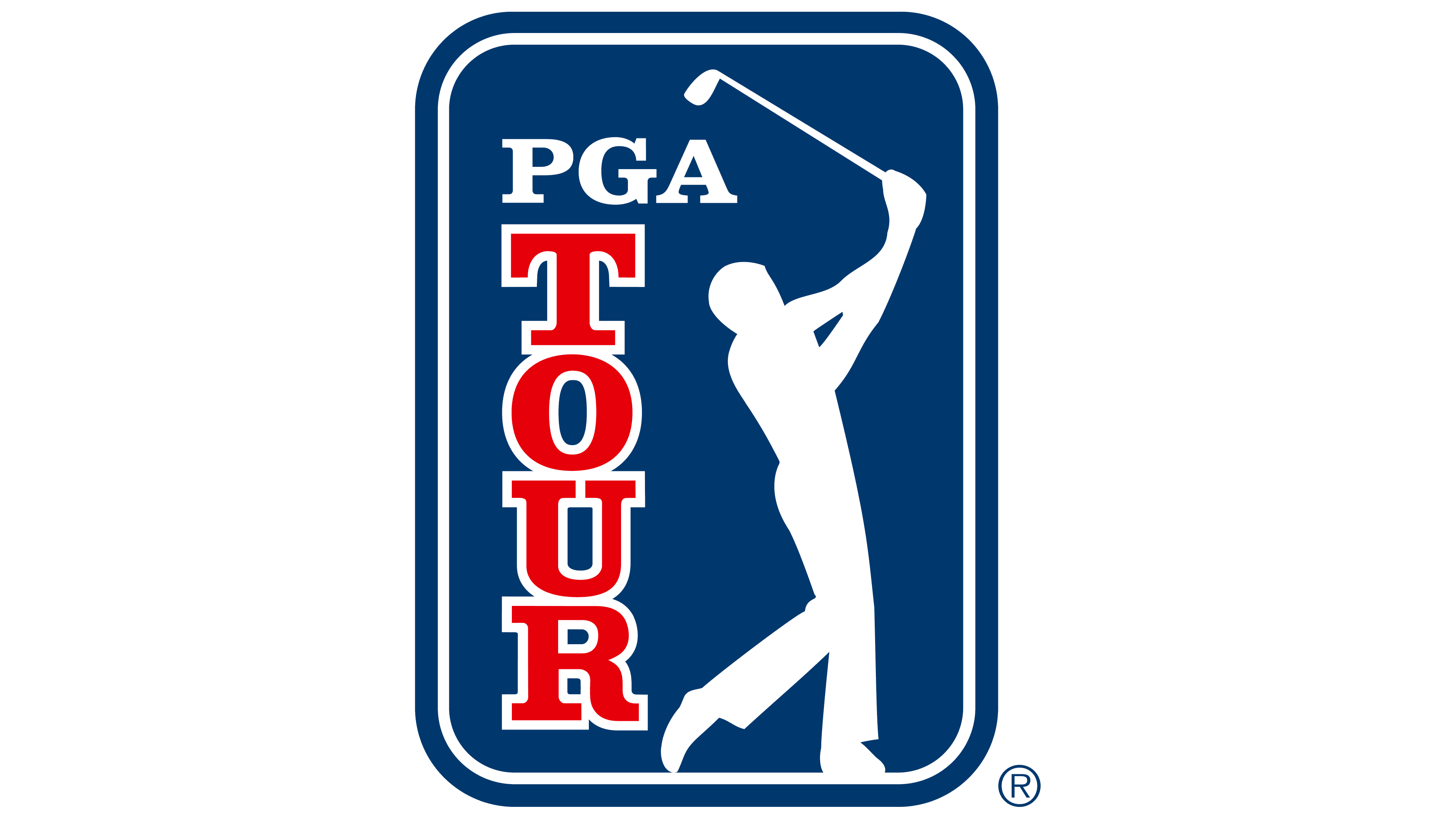
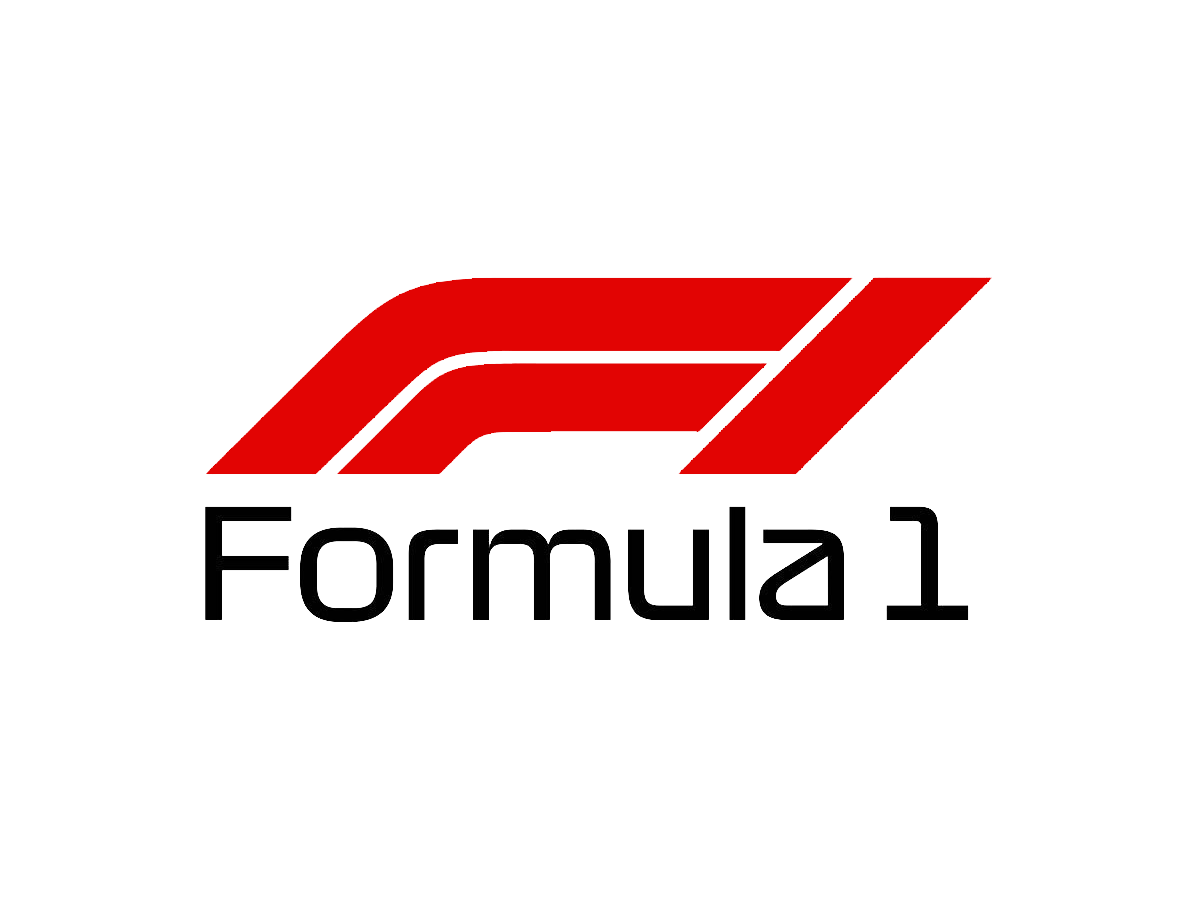
Learn more about AWS